Check out our latest work! ACFR researchers will be presenting the following papers and keynote at the flagship robotics conference IEEE International Conference on Robotics and Automation (ICRA 2023), to be held in London May 29 – June 2:
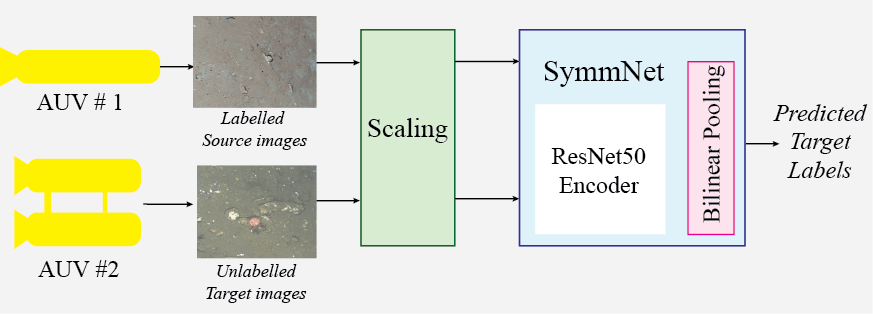
Improving benthic classification using resolution scaling and SymmNet Unsupervised Domain Adaptation
Heather Doig, Oscar Pizarro and Stefan Williams
Autonomous Underwater Vehicles (AUVs) are used to conduct regular photographic surveys of marine environments to characterise and monitor the composition and diversity of the benthos. Using machine learning classifiers for this task is limited due to the low numbers of annotations available and the fine-grained classes involved. Added to this complexity, there is a domain shift between images taken during different AUV surveys due to changes in camera systems, imaging altitude, illumination and water column properties leading to a drop in classification performance for images from a different survey where some or all these elements may have changed. This paper proposes a framework to improve the performance of a benthic morphospecies classifier when used to classify images from a different survey compared to the training data. Using Unsupervised Domain Adaptation with an efficient bilinear pooling layer and image scaling to normalise spatial resolution, improved classification accuracy can be achieved. Our approach was tested on two datasets with images from AUV surveys with different imaging payloads and locations. The results show that a significant increase in accuracy can be achieved for images from an AUV survey that differs from the training images when using the proposed framework.
Preprint: https://arxiv.org/abs/2303.10960
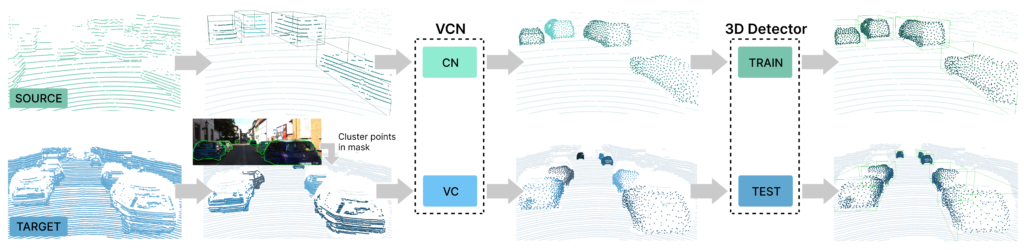
Viewer-Centred Surface Completion for Unsupervised Domain Adaptation in 3D Object Detection
Darren Tsai, Julie Stephany Berrio Perez, Mao Shan, Eduardo Nebot, Stewart Worrall
Every autonomous driving dataset has a different configuration of sensors, originating from distinct geographic regions and covering various scenarios. As a result, 3D detectors tend to overfit the datasets they are trained on. This causes a drastic decrease in accuracy when the detectors are trained on one dataset and tested on another. We observe that lidar scan pattern differences form a large component of this reduction in performance. We address this in our approach, SEE-VCN, by designing a novel viewer-centred surface completion network (VCN) to complete the surfaces of objects of interest within an unsupervised domain adaptation framework, SEE [1]. With SEE-VCN, we obtain a unified representation of objects across datasets, allowing the network to focus on learning geometry, rather than overfitting on scan patterns. By adopting a domain- invariant representation, SEE-VCN can be classed as a multi- target domain adaptation approach where no annotations or re-training is required to obtain 3D detections for new scan patterns. Through extensive experiments, we show that our approach outperforms previous domain adaptation methods in multiple domain adaptation settings.
Preprint: https://arxiv.org/abs/2209.06407
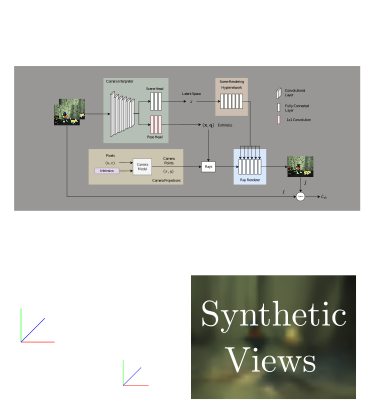
NOCaL: Calibration-Free Semi-Supervised Learning of Odometry and Camera Intrinsics
Ryan Griffiths, Jack Naylor, Donald G. Dansereau
There are a multitude of emerging imaging technologies that could benefit robotics. However the need for bespoke models, calibration and low-level processing represents a key barrier to their adoption. In this work we present NOCaL, Neural odometry and Calibration using Light fields, a semi-supervised learning architecture capable of interpreting previously unseen cameras without calibration. NOCaL learns to estimate camera parameters, relative pose, and scene appearance. It employs a scene-rendering hypernetwork pretrained on a large number of existing cameras and scenes, and adapts to previously unseen cameras using a small supervised training set to enforce metric scale. We demonstrate NOCaL on rendered and captured imagery using conventional cameras, demonstrating calibration-free odometry and novel view synthesis. This work represents a key step toward automating the interpretation of general camera geometries and emerging imaging technologies.
Preprint: https://protect-au.mimecast.com/s/SJ-ICK1DvKTD2ZVxyhMBq-r?domain=arxiv.org