Stephany Berrio Perez has successfully completed her PhD thesis, entitled “Scene understanding and map maintenance for autonomous vehicles applications”. The work was supervised by Professor Eduardo Nebot and Dr Stewart Worrall.
Congratulations Stephany!
Two of the main challenges to enable vehicles to operate autonomously in urban streets are scene understanding and long-term localisation. This thesis addresses both of these topics. A probabilistic sensor data fusion approach is presented to improve the comprehension of the surroundings. A pipeline is presented to maintain a localisation map, ensuring that it remains up to date by adding and removing elements as the environment changes.
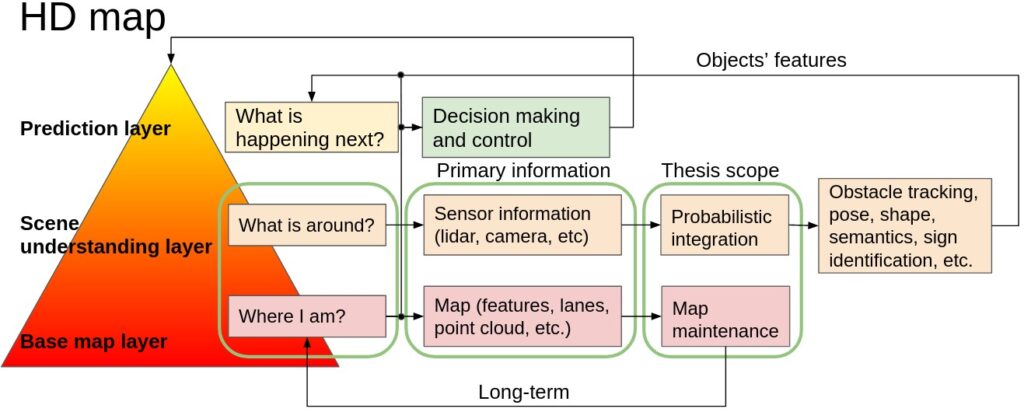
In order to plan and execute accurate and sophisticated driving maneuvers, a high-level contextual understanding of the surroundings is essential. Due to the recent progress in image processing, it is now possible to obtain high definition semantic information in 2D from monocular cameras. However, cameras cannot easily provide the highly accurate 3D information provided by lasers. The fusion of these two sensor modalities can overcome the shortcomings of each sensor. Nevertheless, several critical challenges need to be addressed in a probabilistic manner. This thesis addresses the common, yet challenging, lidar/camera/semantic fusion problems which are seldom approached in a wholly probabilistic manner. This approach is capable of using a multi-sensor platform to build a three-dimensional semantic voxelised map that considers the uncertainty of all processes involved.
This thesis presents a probabilistic pipeline that incorporates uncertainties from the sensor readings (cameras, lidar, IMU and wheel encoders), compensation for the motion of the vehicle, and heuristic label probabilities for the semantic images. It also includes a novel and efficient viewpoint validation algorithm to check for occlusions from the camera frames. A probabilistic projection is performed from the camera images to the lidar point cloud. For testing purposes, each labelled lidar scan then feeds into an octree map building algorithm that updates the class probabilities of the map voxels every time a new observation is available. This approach is validated using a set of qualitative and quantitative experimental tests. These tests demonstrate the usefulness of a probabilistic sensor fusion approach by evaluating the performance of the perception system in a typical autonomous vehicle application.
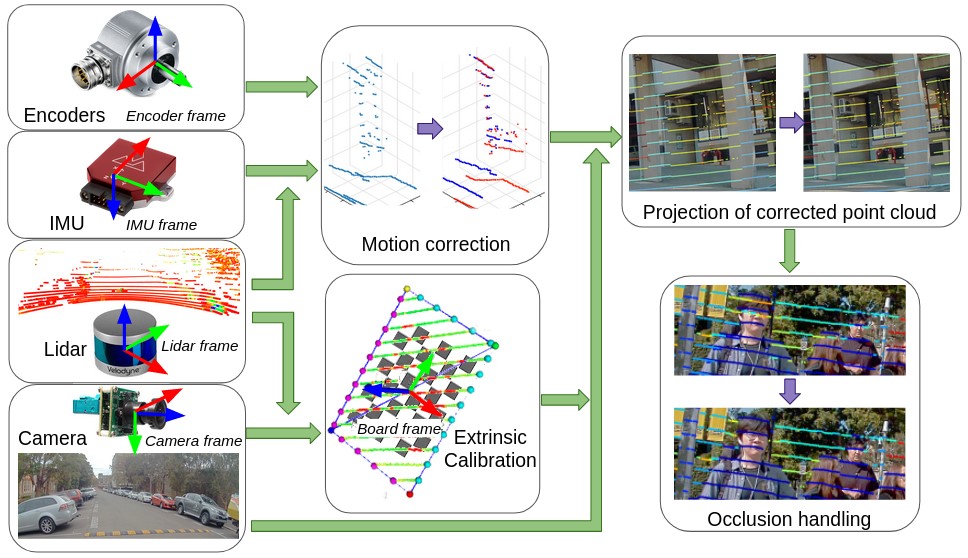
For autonomous vehicles to operate persistently in a typical urban environment, it is essential to have high accuracy position information. This requires a mapping and localisation system that can adapt to changes over time. A localisation approach based on a single-survey map will not be suitable for long-term operation as it does not account for variations in the environment over time. This thesis presents new algorithms to maintain a featured-based map. A map maintenance pipeline is proposed that can continuously update a map with the most relevant features taking advantage of the changes in the surroundings. The proposed pipeline detects and removes transient features based on their geometric relationships with the vehicle’s pose. Newly identified features became part of a new feature map and are assessed by the pipeline as candidates for the localisation map. By purging out-of-date features and adding newly detected features, we continually update the prior map to represent the most recent environment more accurately. The algorithms presented in this thesis can only be validated with data taken over a long period of time and in environments with significant structural variations during this period.
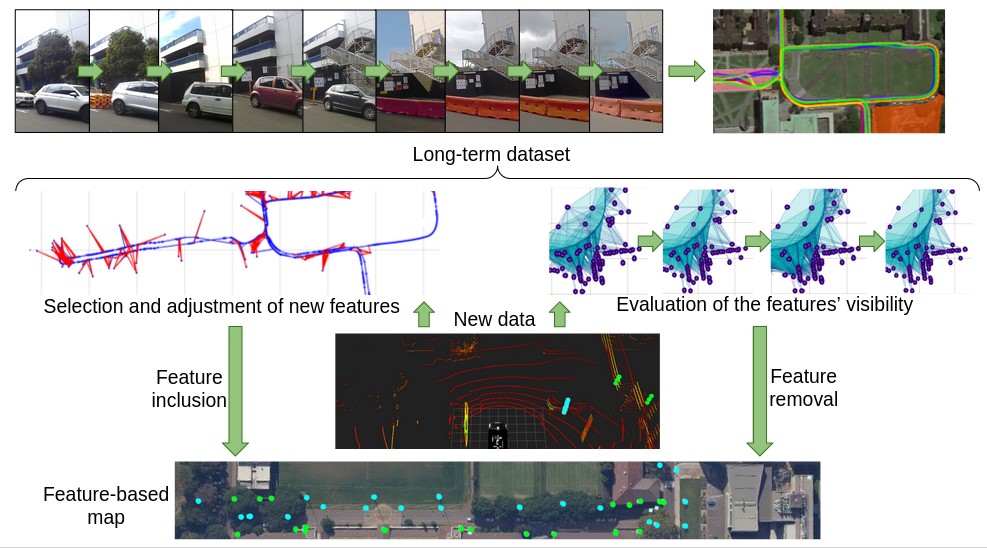
This thesis introduces large-scale and long-term dataset named “The Usyd Campus Dataset“. An electric vehicle equipped with multiple sensors logged proprioceptive and external data. The vehicle was driven around the University of Sydney campus weekly for more than a year. The dataset covers local information which consists of diverse illumination conditions, seasonal variations, structural changes and various traffic (vehicles/pedestrians) volumes. The map maintenance pipeline was validated using this dataset. The results presented demonstrate that the proposed approach produces a resilient map that can provide sustained localisation performance over time.
Dataset video: https://www.youtube.com/watch?v=9ANpC1nFtC8
This video present a long-term large-scale dataset collected for the period of one year on a weekly basis at the University of Sydney surroundings.