When: Thursday 24th of February, 1pm AEDT
Where: This seminar will be partially presented at the Rose Street Seminar area (J04) and partially online via Zoom, RSVP here.
Speaker: James Allworth
Title: A Machine Learning Approach to Space Debris Characterisation and Classification
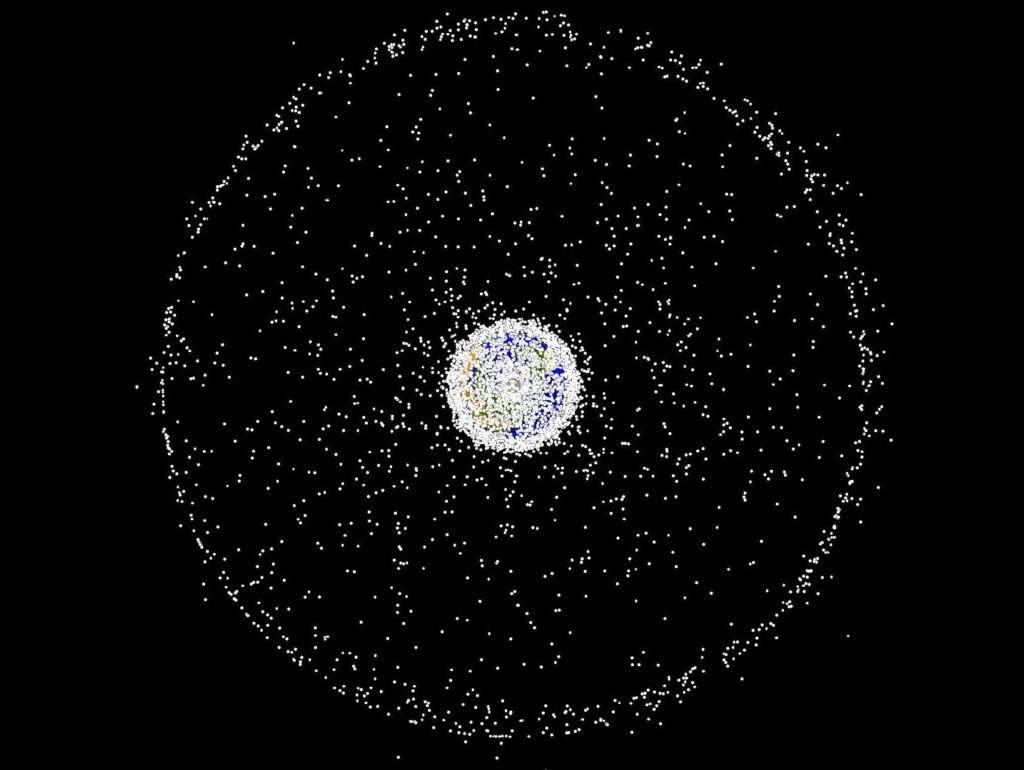
Abstract:
Space debris is becoming an increasingly prevalent issue through a combination of the recent rise in the accessibility of space and the current difficulty in actively removing space debris from orbit. As a result of the high relative velocity between orbital objects and the difficulty in accurately maintaining the state of all objects, space debris poses a significant collision risk to active satellites. Current risk mitigation strategies rely on space situational awareness, which focuses on tracking space objects and then using orbital mechanics to predict their future states. Satellite operators can then be informed of any potential future conjunctions between debris and active satellites. For this method to be effective, the information provided to satellite operators must be both accurate and timely. The accuracy of these predictions is limited by a lack of knowledge about the physical characteristics of a large proportion of space debris, such as shape, size, orientation, mass and material properties.
This thesis outlines a data-driven approach to space object characterisation through the application of neural networks to light curves extracted from non-resolved ground based optical observations. A light curve is a temporal history of an object’s brightness and has been shown to contain information about the object’s physical characteristics. Neural networks are known to be more effective when they are trained on a well-labelled dataset with a large number of examples in order for the complex non- linear relationships within the data to be learned. This has previously been a limiting factor when applying deep learning to light curve based object classifications as light curves are difficult to obtain and label, so real world datasets remain relatively small.
This thesis presents simulation-based transfer learning as a method for overcoming this limitation and improving shape classification performance on real world light curve datasets. A high-fidelity light curve simulation environment has been developed in order to generate a large, well-labelled simulated light curve dataset that encapsulates the features present in real world light curves. The simulated dataset is used for the initial training of a one dimensional convolutional neural network. This pre-trained model is then transferred to the smaller real world light curve dataset where it is fine-tuned. This process is shown to boost the performance of the model on the real world dataset, compared to models that are trained only on the real world dataset. To further improve performance on challenging cases, a framework for effectively combining multiple light curve observations of a single object is also developed. Finally, in order to utilise this framework efficiently, a targeted scheduling process has been developed, using uncertainty quantification of the neural network output, to selectively prioritise the re-observation of challenging cases and thus reduce misclassifications.
Bio: James Allworth is currently a postdoc researcher at the ACFR working on automated harvest yield estimation for commercial pine forests using LIDAR scans. James recently submitted his PhD thesis, which focused on space debris characterisation from ground-based optical observations using a data driven approach. This research was conducted in collaboration with industry partner Electro Optic Space Systems, where James previously worked as an astrodynamics researcher.