When: Thursday 19th of Sept, 1:00pm AEST
Where: This seminar will be partially presented at the ACFR seminar area, J04 lvl 2 (Rose St Building) and partially online via Zoom. RSVP
Speaker: Ziting Wen
Title: Label-Efficient Deep Learning with the Pre-trained Models
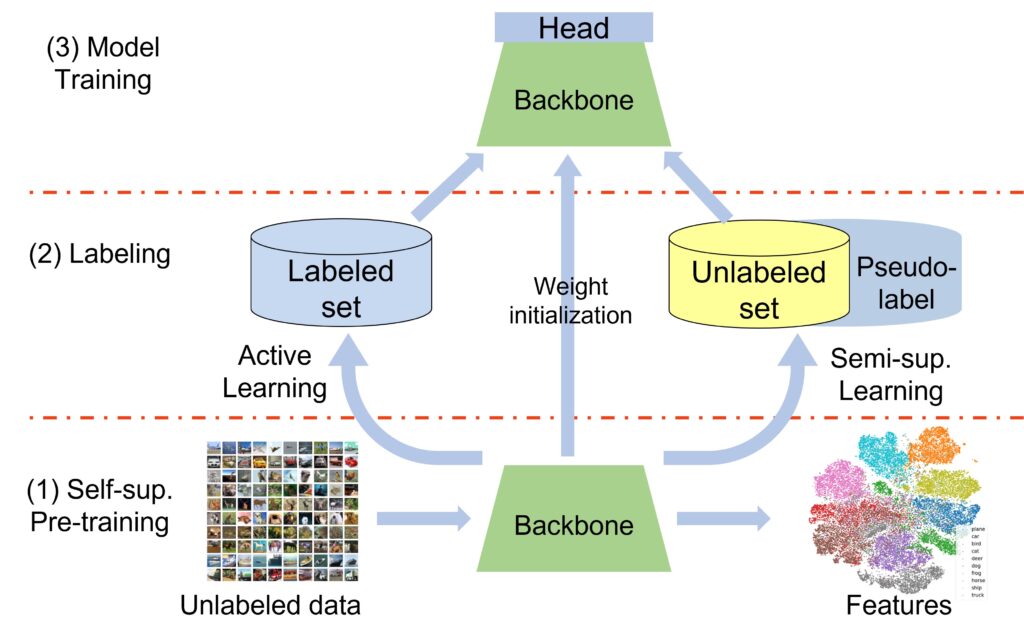
Abstract:
The power of deep neural networks stems from large-scale data, but collecting vast amounts of data—especially labeled data—is both costly and labor-intensive. Constructing informative labeled datasets through active learning and leveraging unlabeled data via semi-supervised learning are key methods to address this issue. However, these approaches face bottlenecks in reducing the need for labeled samples because they typically require a substantial labeled data to train an effective initial model. For example, active learning relies on a well-trained model to identify informative samples, while semi-supervised learning depends on a good model to generate high-quality pseudo-labels for unlabeled data.
Recent advances in pre-training have alleviated some of this burden by enabling models to learn valuable features without annotations. In this seminar, I will discuss the challenges of implementing active learning and semi-supervised learning on top of pre-trained models, such as phase transitions of active learning and high training costs. I will also present our studies, which improve the effectiveness of active learning using Neural Tangent Kernel (NTK) and pseudo-labels, enhance computational efficiency via proxy models, and boost the performance of semi-supervised training in scenarios with few labeled data by guiding the process with prior pseudo-labels.
Bio:
Ziting Wen received his Master’s degree in information and communication engineering from Shanghai Jiao Tong University, China in 2020. Since then, he has been a PhD student in the marine robotics group, ACFR. His research interests include active learning and semi-supervised learning.