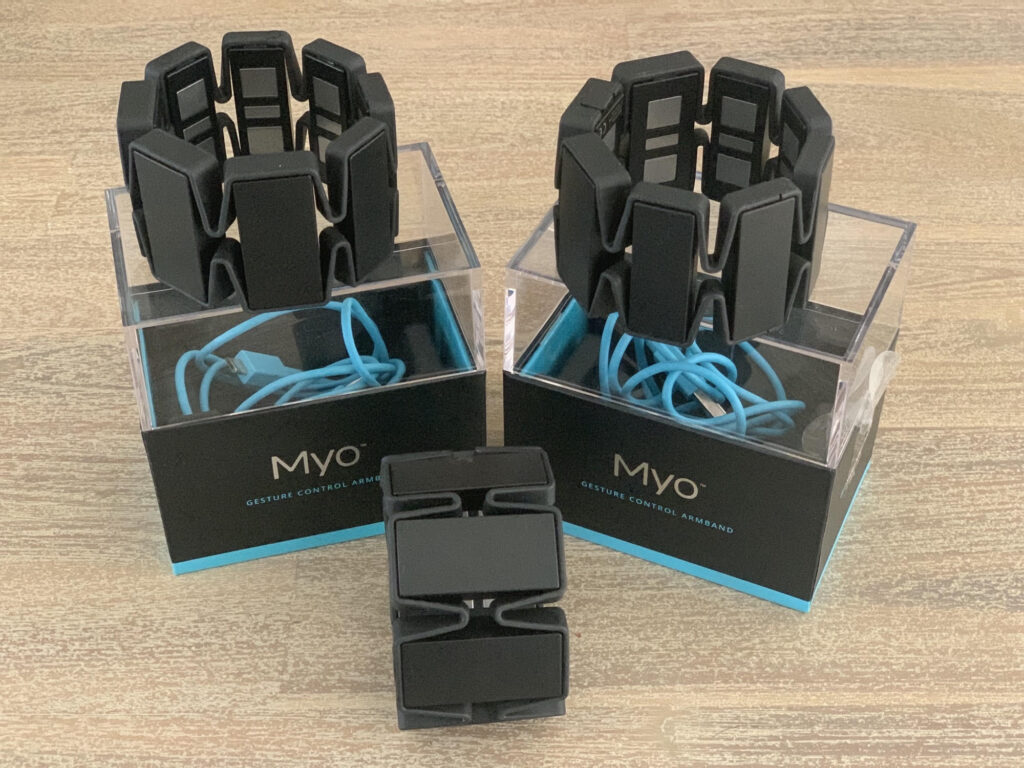
When: Thurs 17th of Sept, 1p AEST
Where: This seminar will be presented online, RSVP here.
Speaker: Dr Rami N. Khushaba, PhD, M.Sc., B.Sc., Senior Member – IEEE
Title: Myoelectric Pattern Recognition Using Recursive Spatial-Temporal Fusions: Building your own deep features representations
Abstract: The design of pattern recognition-based neural interfaces has been heavily explored and contested in the research literature. A considerable proportion of the performance of these interfaces has been linked to the quality of the feature extraction (FE) stage used to describe the underlying Electromyogram (EMG) signal. In this presentation, we address two important factors of FE that have not been fully exploited; 1) Spatial focus – traditional FE methods focus mainly on the concatenation of features extracted from individual channels/sensors and 2) Temporal focus – available FE methods are cross-sectional in nature, largely ignoring the temporal information that may exist between feature windows. To overcome these limitations, several spatiotemporal FE methods have been proposed including hand-crafted and deep learning (DL) models, with the latter showing significant performance enhancements at the cost of increased computational burden. Consequently, rather than applying DL directly, this presentation will guide you on how to leverage concepts derived from these models to build upon our proposed concept of a Fusion of Time Domain Descriptors (FTDD). The FTDD are augmented with Range Spatial Filtering (RSF) to capture the spatial correlations and combined into a Long Short-Term Memory (LSTM)-style framework. This process, denoted as Recursive Spatial-Temporal Fusion (RSTF), can be applied in combination with any traditional feature extraction method to exploit temporal and spatial correlations, with the potential for bi-directional applications. The advantages of the proposed RSTF method include 1) the memory concept, capturing long- and short-term spatial and temporal dependencies of the EMG signals, 2) significantly improved performance outperforming other state-of-the-art models and 3) the simplicity and low computational costs with which the features are extracted. Experiments on more than 65 intact-limbed and amputee subjects reveal a substantial reduction in classification errors as compared to models built with other feature sets.
Bio: Rami finished his PhD degree at UTS (Centre for Intelligent Mechatronic Systems-2010), with a focus on the neural control of powered prosthetics using the Electromyogram (muscles) & Electroencephalogram (brain) signals. As a postdoc at UTS, he worked on several projects related to driver drowsiness/fatigue detection, decision making and neuromarketing, and controlling exoskeletons (2010-2012). He joined ResMed Ltd. as an Algorithms Development Engineer working on several applications related to Sleep-Disordered Breathing (SDB) detection using Radar & other signals from Heart failure and COPD patients (2012-2017). He joined BuildingIQ as a Senior Data Scientist and then Team Lead/Data-Science working on energy optimization, analytics, modelling and verification and causal-impact based fault detection in HVAC systems in large commercial buildings (2017-2020). He joined the Rio Tinto Centre for Mine Automation (RTCMA) as a Theme lead for Equipment Automation in Feb 2020 and is currently working on several projects within this theme.