When: Thursday 30th of May, 1:00pm AEST
Where: This seminar will be partially presented at the ACFR seminar area, J04 lvl 2 (Rose St Building) and partially online via Zoom. RSVP
Speaker: Prof Ian Manchester
Title: Robust Learning for Dynamics, Control, and Optimization
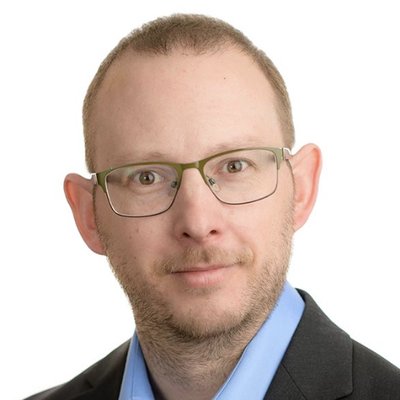
Abstract:
In this talk, we will introduce a new approach to building neural networks models, both static (feedforward) and dynamic (recurrent), with built-in guarantees of stability, robustness, invertibility and other desirable properties. We will trace the connections between convex parameterizations from robust control to so-called “direct” parameterizations, i.e. smooth & unconstrained parameterisations of all models that satisfy prescribed conditions. These direct parameterizations enable learning of robust static and dynamic models via simple first-order methods, without any auxiliary constraints or projections. We will explore some applications in certifiably-robust image classification, physics-informed learning of nonlinear observers, robust adaptive control, and learning of “easy-to-optimize’’ surrogate loss functions.
Bio:
Ian R. Manchester received the B.E. (Hons 1) and Ph.D. degrees in electrical engineering from the University of New South Wales, Sydney, NSW, Australia, in 2002 and 2006, respectively. From 2006-2009 he was a post-doctoral researcher at Umea University, Sweden, and from 2009-2012 he was a Research scientist at the Massachusetts Institute of Technology. Since 2012 he has been a faculty member at the University of Sydney, Australia, where he is currently Professor of Mechatronic Engineering, Director of the Australian Centre for Robotics, and Director of the Australian Robotic Inspection & Asset Management Hub. His current research focuses on algorithms for control, estimation, and learning of nonlinear dynamical systems, with applications in robotics, robust machine learning, and other fields.