When: Tuesday 3rd of November, 1pm AEDT
Where: This seminar will be partially presented at the Rose Street Seminar area (J04) and partially online via Zoom, RSVP here.
Speaker: Patricia Pauli (University of Stuttgart)
Title: Robustness certification and training of robust neural networks using Lipschitz bounds
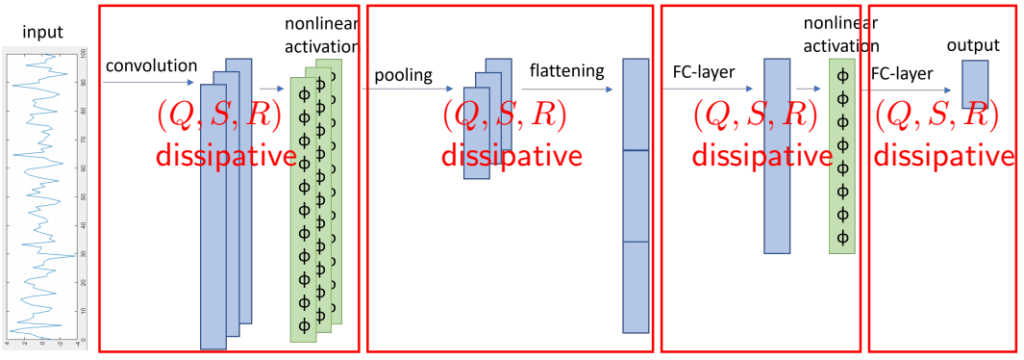
Abstract:
Due to their susceptibility to adversarial perturbations, neural networks (NNs) are hardly used in safety-critical applications. One measure of robustness to such perturbations in the input is the Lipschitz constant of the input-output map defined by an NN. In this talk, we address the topics of Lipschitz certification and the synthesis of Lipschitz bounded neural networks. First, we consider fully connected neural networks and over-estimate the underlying nonlinear activation functions by incremental quadratic constraints using that they are slope restricted. Using this, we propose a training method for Lipschitz-bounded NNs based on interior point methods. Further exploiting the block tridiagonal structure of the underlying matrix constraint, we set up an efficient and scalable training scheme for NN training. In the second part of the talk, we address Lipschitz certification of 1D convolutional neural networks. To certify the Lipschitz constant from the input to the output, we connect the individual layers through their dissipativity properties. We introduce incremental quadratic constraints to describe pooling layers and we formulate convolutional layers compactly in state space, yielding a scalable method for Lipschitz certification of 1D convolutional neural networks.
Bio:
Patricia Pauli is a PhD student with the Institute for Systems Theory and Automatic Control at the University of Stuttgart under the supervison of Prof. Frank Allgöwer, who is currently visiting the ACFR for three months. She received two M.Sc. degrees in Mechanical Engineering and Computational Engineering, respectively, in 2019. She spent two semesters of her M.Sc. studies at the University of California, Berkeley and carried out her M.Sc. thesis at Massachusetts Institute of Technology. Her research interests are in the area of robust machine learning and learning based control.