When: Thursday 29th of June, 1pm AEST
Where: This seminar will be partially presented at the Rose Street Seminar area (J04) and partially online via Zoom. RSVP
Speaker: Jack Naylor
Title: Smoke & Mirrors: Neural Scene Representations for Robotics
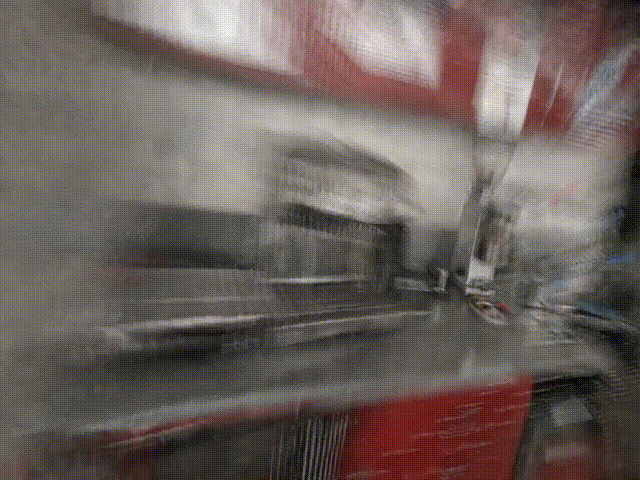
Abstract:
Accurate representation of a robot’s surroundings is critical to understanding dangers and performing given tasks safely and reliably. Complex appearances due to reflection and transparency are significant challenges to traditional perception suites, particularly in urban, polar and near water environments. These hinder feature-based visual perception pipelines and provide spurious depth measurements from modalities such as LiDAR, reducing the accuracy of scene representation. Neural radiance fields (NeRFs) are a representation which can perform realistic novel view synthesis owing to a view-dependent appearance, thereby allowing these visual phenomena to be represented. Whilst NeRF has strong visual fidelity, a robotic map representation needs accurate geometry to perform downstream tasks, like localisation, accurately. This talk will introduce key concepts in neural scene representations in the context of robotics and introduce novel regularisation techniques to produce geometrically accurate NeRFs as a key step towards localising and mapping in neural worlds.
Bio:
Jack Naylor is a second year PhD candidate in the Robotic Imaging group supervised by Dr. Donald Dansereau, with the Australian Centre for Robotics at the University of Sydney, Australia. He received his Bachelor of Engineering (Mechanical) (Hons. I) and Bachelor of Science (Advanced) degrees from the University of Sydney in 2022 where he majored in Space Engineering and Physics. His research seeks to adapt neural representations of light, in the form of neural radiance fields (NeRFs), as a new robotic map representation to enable understanding of complex visual phenomena in unstructured environments. His research interests include robotic perception, computational imaging, remote sensing and neural implicit representations, with particular application to the space domain.