When: Thursday 5th of Sept, 1:00pm AEST
Where: This seminar will be partially presented at the J18 lvl 4 Conference Room and partially online via Zoom. RSVP
Speaker: Dr Jesse Mehami
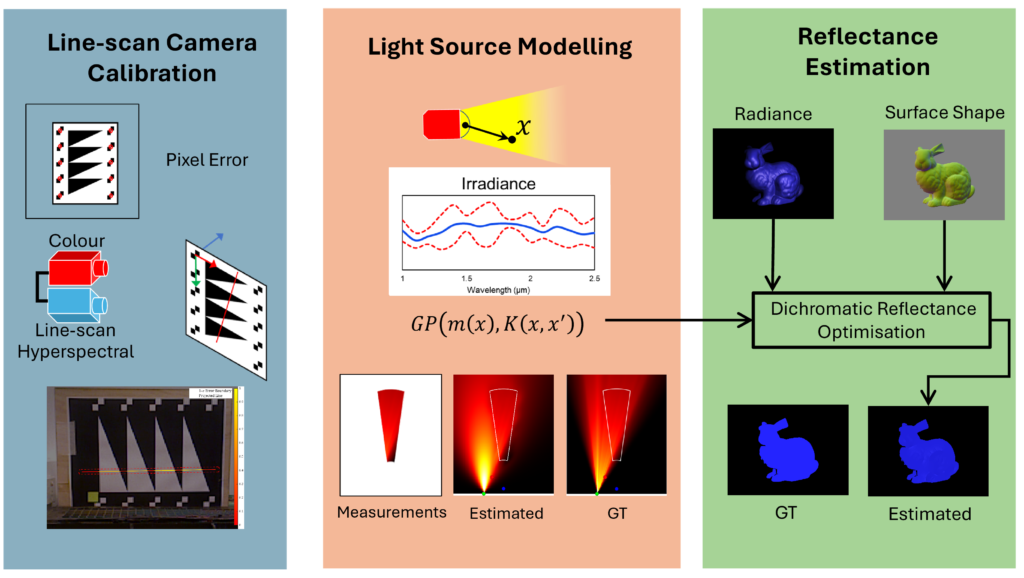
Title: Subsurface Material Estimation using Hyperspectral Imaging
Abstract:
Traditional vision relies on visible light perception, limiting the features detectable within materials without invasive techniques. Beyond visible light has the potential to penetrate surfaces, and hyperspectral imaging is one technology capable of measuring it. Modelling features within an object requires proper estimation of the captured material, which is an inverse problem that is heavily underconstrained due to unknown lighting, shape and perspective. A multi-modal vision system incorporating an RGB-D camera improves spatial understanding from hyperspectral images so that perspective can be determined. Still, Illumination on the object varies in both intensity and direction. The Illumination can be accurately estimated on the object’s surface by prior light source modelling. The hyperspectral camera measures radiance, which is influenced by Illumination, shape, and the respective material. Reflectance is an intrinsic material property, which can be estimated from radiance by assuming that the material’s behaviour with light follows a particular model. Given that shape can be easily acquired through 3D reconstruction and prior modelling of the light source, an optimisation is devised that uses this prior knowledge to improve recovery of reflectance. This work is successfully applied to modelling the subcutaneous fat on lamb carcasses using deep learning.
Bio:
Jasprabhjit Mehami obtained his PhD from the Robotics Institute (RI) at the University of Technology Sydney (UTS), where he worked on non-invasively estimating subcutaneous fat layer thickness on lamb carcasses through hyperspectral imaging. His experience at UTS involved projects working with multi-camera systems, during which time he devised computer vision algorithms to manipulate and understand different imaging modalities. His time at UTS was concluded as a research engineer focusing more on deep learning methods for his previous research. Before his PhD, he graduated from The University of Auckland (UOA) with a bachelor of engineering honours degree, specialising in Mechatronics. His work at the time demonstrated RFID technologies for smart factories towards innovations in Industry 4.0.
His current research interests lie in computer vision, machine learning, and computational imaging, leading to his current position as a Postdoc on the Smartsat In-orbit Servicing, Assembly and Manufacturing (ISAM) project. This project aims to demonstrate autonomous in-orbit maintenance of satellites, where his research will focus on developing novel imaging methods for capturing improved images of satellites in space.