Check out our latest work! ACFR researchers will be presenting the following papers and keynote at the flagship robotics conference IEEE International Conference on Robotics and Automation (ICRA 2022), to be held in Philadelphia May 23 – 27:
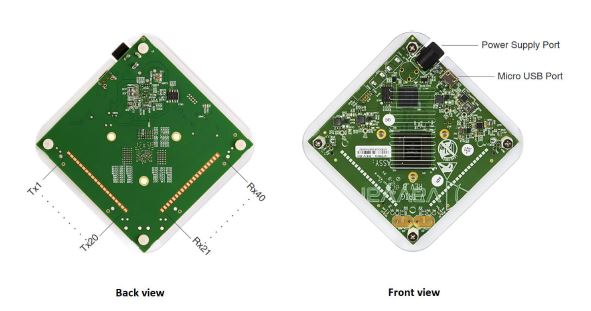
Radar-based Materials Classification Using Deep Wavelet Scattering Transform: A Comparison of Centimetre vs. Millimetre Wave Units
Rami N. Khushaba, Andrew J. Hill
Radar-based materials detection received significant attention in recent years for its potential inclusion in consumer and industrial applications like object recognition for grasping and manufacturing quality assurance and control. Several radar publications were developed for material classification under controlled settings with specific materials’ properties and shapes. Recent literature has challenged the earlier findings on radars-based materials classification claiming that earlier solutions are not easily scaled to industrial applications due to a variety of real-world issues. Published experiments on the impact of these factors on the robustness of the extracted radar-based traditional features have already demonstrated that the application of deep neural networks can mitigate, to some extent, the impact to produce a viable solution. However, previous studies lacked an investigation of the usefulness of lower frequency radar units, specifically <10GHz, against the higher range units around and above 60GHz. This research considers two radar units with different frequency ranges: Walabot-3D (6.3-8 GHz) cm-wave and IMAGEVK-74 (62-69 GHz) mm-wave imaging units by Vayyar Imaging. A comparison is presented on the applicability of each unit for material classification. This work extends upon previous efforts, by applying deep wavelet scattering transform for the identification of different materials based on the reflected signals. In the wavelet scattering feature extractor, data is propagated through a series of wavelet transforms, nonlinearities, and averaging to produce low-variance representations of the reflected radar signals. This work is unique in comparison of the radar units and algorithms in material classification and includes real-time demonstrations that show strong performance by both units, with increased robustness offered by the cm-wave radar unit.
Preprint: https://arxiv.org/abs/2202.05169
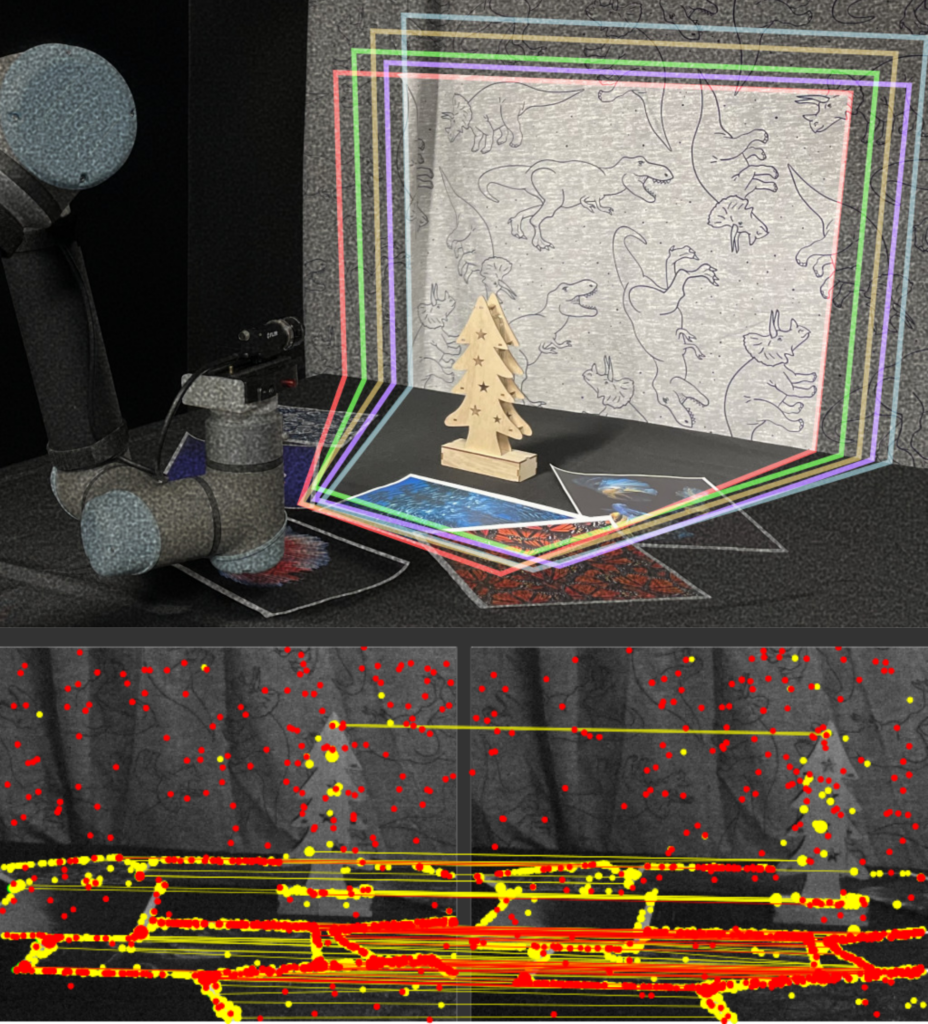
Burst Imaging for Light-Constrained Structure-From-Motion
Ahalya Ravendran, Mitch Bryson, and Donald G. Dansereau
Images captured under extremely low light conditions are noise-limited, which can cause existing robotic vision algorithms to fail. In this paper we develop an image processing technique for aiding 3D reconstruction from images acquired in low light conditions. Our technique, based on burst photography, uses direct methods for image registration within bursts of short exposure time images to improve the robustness and accuracy of feature-based structure-from-motion (SfM). We demonstrate improved SfM performance in challenging light-constrained scenes, including quantitative evaluations that show improved feature performance and camera pose estimates. Additionally, we show that our method converges more frequently to correct reconstructions than the state-of-the-art. Our method is a significant step towards allowing robots to operate in low light conditions, with potential applications to robots operating in environments such as underground mines and night time operation.
Preprint, dataset and code: https://roboticimaging.org/Projects/BurstSfM/
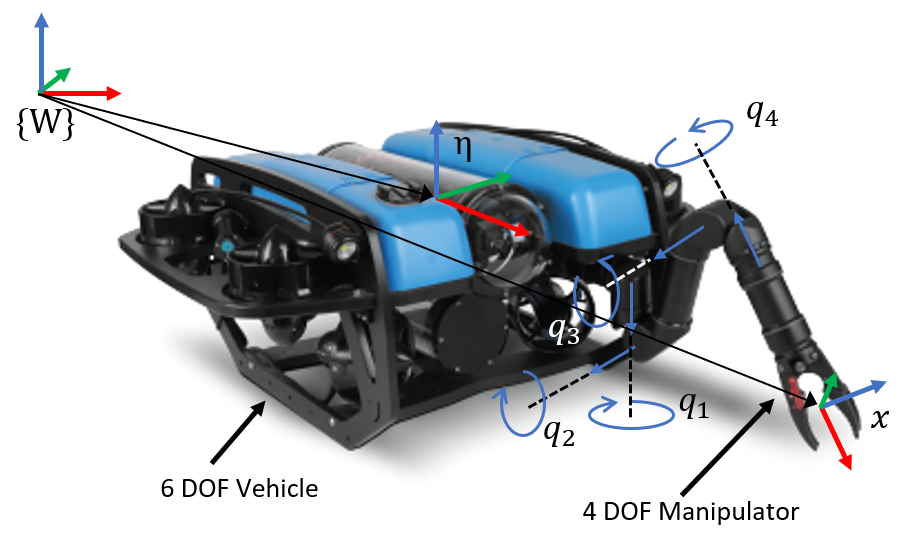
Go With the Flow: Energy Minimising Periodic Trajectories for UVMS
Wilhelm Johan Marais, Stefan B. Williams, Oscar Pizarro
For Underwater Vehicle Manipulator Systems (UVMS), the ability to keep a fixed end effector pose is required for intervention tasks. Maintaining a static configuration in a dynamic underwater environments requires significant amounts of energy over time, limiting the operational time for battery powered systems. In this work we consider learning the periodic components of the dynamic flow in order to generate periodic trajectories which keep the end effector fixed, yet minimise the energy expenditure over time. We compare this proposed ‘go with the flow’ approach to the static configuration case for a fixed end effector pose, and show a significant reduction in energy use.
Preprint: https://robotics.sydney.edu.au/wp-content/uploads/2022/02/marais2020flow_preprint.pdf
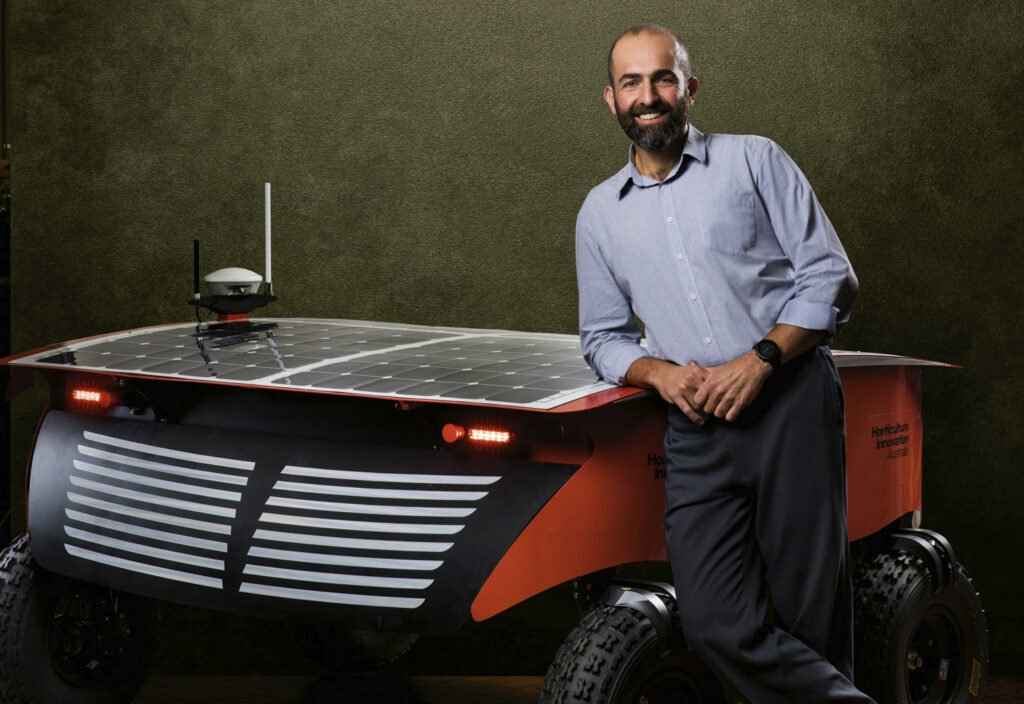
Keynote Speaker: Salah Sukkarieh
ICRA 2022 Keynote Speakers: https://www.icra2022.org/program/plenary-keynote-speakers
Salah’s Profile: https://sydney.edu.au/engineering/people/salah.sukkarieh.php